Research on Integrated Digital Twins and Predictive Maintenance - Eureka
Digital Twins and Predictive Maintenance Goals
Tracing the evolution of these technologies, highlighting key milestones and breakthroughs that have shaped their progress. Analyzing the current technological landscape, identifying major challenges and constraints that need to be addressed. Exploring potential future directions and innovative solutions that could drive further advancements in this field, based on emerging research and industry trends.
Market Demand for Digital Twins and Predictive Maintenance
- Market Size and Growth
The global market for digital twins and predictive maintenance solutions is rapidly expanding, driven by the increasing adoption of Industry 4.0 technologies and the need for optimized asset management. According to industry reports, the market is projected to grow at a CAGR of over 35% during the forecast period, reaching a value of over $50 billion by 2027. - Industry Demand Drivers
The demand for digital twins and predictive maintenance is fueled by several factors:- Need for improved asset utilization and reduced downtime
- Increasing emphasis on operational efficiency and cost optimization
- Adoption of IoT and advanced analytics for real-time monitoring
- Regulatory compliance and safety requirements in critical industries
- Key Application Areas
- Manufacturing: Optimizing production processes, reducing maintenance costs, and improving product quality
- Energy and Utilities: Monitoring and maintaining critical infrastructure, improving asset reliability, and reducing energy consumption
- Transportation: Predictive maintenance of vehicles, aircraft, and transportation systems
- Healthcare: Monitoring and maintaining medical equipment, optimizing facility operations
- Regional Market Dynamics
The adoption of digital twins and predictive maintenance solutions varies across regions, with North America and Europe being the early adopters, followed by the Asia-Pacific region. Factors such as industrial automation, technological advancements, and regulatory frameworks influence regional market dynamics.
Current State and Challenges of Digital Twins
- Widespread Adoption
Digital twins have gained widespread adoption across various industries, enabling virtual representations of physical assets, processes, and systems. - Technological Advancements
Advancements in IoT, big data analytics, and AI/ML have facilitated the development of more sophisticated and accurate digital twins. - Integration Challenges
Integrating data from disparate sources, ensuring data quality, and establishing seamless communication between physical and virtual entities remain challenges. - Computational Complexity
Modeling complex systems and simulating intricate interactions in real-time pose computational challenges, requiring powerful hardware and software solutions. - Cybersecurity Risks
As digital twins handle sensitive data and control critical systems, robust cybersecurity measures are crucial to mitigate risks and ensure data integrity. - Standardization Efforts
Efforts are underway to establish industry standards and best practices for digital twin development, deployment, and interoperability.
Evolution of Digital Twin Technologies
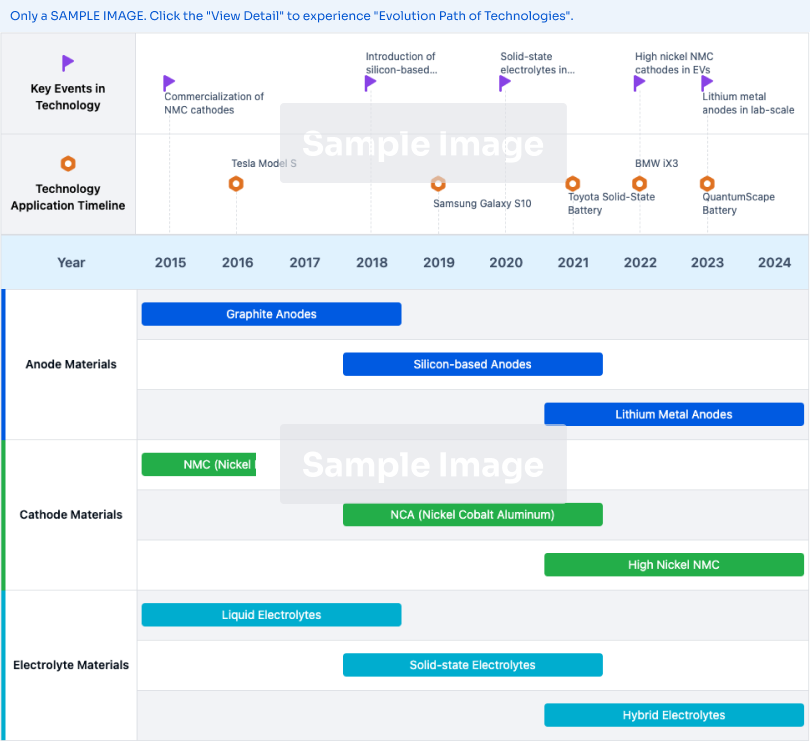
Existing Solutions for Predictive Maintenance
01 Digital Twin Models for Predictive Maintenance
Creating digital twin models of physical assets or systems to predict maintenance or repair needs by simulating behavior and performance, allowing for early issue detection and proactive maintenance planning.- Digital Twin Models for Predictive Maintenance: Creating digital twin models of physical assets or systems to predict maintenance or repair needs by simulating behavior and performance, enabling early issue detection and proactive maintenance planning.
- Digital Twins for Equipment Monitoring: Combining digital twin technology with predictive maintenance strategies to monitor and analyze equipment condition, using digital twins as virtual representations for real-time monitoring, data analysis, and decision-making.
- Edge Computing and Digital Twins: Leveraging edge computing capabilities with digital twins to enable predictive maintenance at the edge, closer to physical assets, allowing real-time data processing and analysis.
- Intelligent Decision-Making with Digital Twins: Using digital twins with intelligent algorithms and decision-making frameworks to optimize predictive maintenance strategies, enabling data-driven decision-making for maintenance planning and execution.
- Scalable Digital Twin Management: Efficient and scalable creation, deployment, and management of digital twins for predictive maintenance applications, addressing challenges related to large-scale data, interoperability, and integration into existing workflows.
02 Digital Twins for Equipment Monitoring
Combining digital twin technology with predictive maintenance strategies to monitor and analyze equipment condition, using digital twins as virtual representations for real-time monitoring, data analysis, and decision-making.03 Edge Computing and Digital Twins
Leveraging edge computing capabilities with digital twins to enable real-time data processing and predictive maintenance decision-making at the edge, closer to physical assets, improving response times and reducing latency.04 Intelligent Systems for Predictive Maintenance
Developing intelligent systems that utilize digital twins as a driving force for predictive maintenance decision-making, incorporating techniques like machine learning, data analytics, and simulation to optimize maintenance strategies and decision-making processes.05 Probabilistic and Multi-Layer Modeling
Using probabilistic modeling and multi-layer modeling approaches to enhance the accuracy and reliability of digital twins in predictive maintenance applications, accounting for uncertainties, capturing complex system behaviors, and providing robust predictions for maintenance planning.
Key Players in Digital Twin and Maintenance Industry
International Business Machines Corp.
Accenture Global Solutions Ltd.
Core Innovations in Digital Twins
- Integrating digital twin technology with deep learning techniques for optimizing industrial production control management
- Leveraging real-time data from sensors and IoT devices for precise predictive modeling and timely decision-making
- Employing advanced deep learning methods like RNNs, CNNs, Autoencoders, GANs, and LSTMs for forecasting equipment breakdowns, optimizing supply chain operations, and enhancing manufacturing processes
Future Directions for Digital Twins and Maintenance
- Hybrid Digital Twins
- Federated Digital Twins
- Explainable Digital Twins