Research on the development and applications of artificial intelligence and machine learning technologies - Eureka
AI and ML Development History and Goals
Additionally, it will clearly define the expected technological goals and capabilities that AI and ML systems aim to achieve, outlining the desired outcomes and potential applications across various domains. By establishing a solid understanding of the historical context and envisioned targets, this section lays the foundation for a thorough analysis of the market demands, technological challenges, and potential innovation pathways in subsequent sections.
Market Demand for AI and ML Applications
- Growing Demand for AI/ML Solutions
The market demand for AI and ML applications is rapidly increasing across various industries, driven by the need for automation, data-driven insights, and improved decision-making processes. - Diverse Application Areas
AI and ML technologies are being adopted in sectors such as healthcare, finance, retail, manufacturing, and transportation, enabling innovative solutions and enhancing operational efficiency. - Personalization and Customer Experience
Businesses are leveraging AI and ML to personalize products, services, and customer experiences, leading to increased customer satisfaction and loyalty. - Predictive Analytics and Forecasting
The ability of AI and ML algorithms to analyze large datasets and identify patterns is driving demand for predictive analytics and forecasting applications in areas like sales, supply chain management, and risk assessment. - Automation and Process Optimization
AI and ML technologies are being adopted to automate repetitive tasks, streamline processes, and optimize resource allocation, resulting in cost savings and improved productivity.
Current State and Challenges in AI and ML
- Rapid Advancement
AI and ML technologies have witnessed rapid advancement in recent years, driven by breakthroughs in deep learning, neural networks, and big data analytics. - Widespread Applications
These technologies have found widespread applications across various domains, including computer vision, natural language processing, robotics, and healthcare. - Computational Challenges
However, the current state of AI and ML still faces significant computational challenges, such as the need for massive computing power, efficient data processing, and scalability. - Data Quality and Bias
Data quality and potential biases in training datasets remain critical issues, which can lead to inaccurate or biased models and decision-making. - Interpretability and Transparency
Many AI and ML models are often perceived as "black boxes," lacking transparency and interpretability, which hinders their adoption in critical decision-making processes. - Ethical and Regulatory Concerns
The rapid development of AI and ML has also raised ethical and regulatory concerns, such as privacy, security, and the potential impact on employment and society.
Evolution Path of AI and ML Technologies
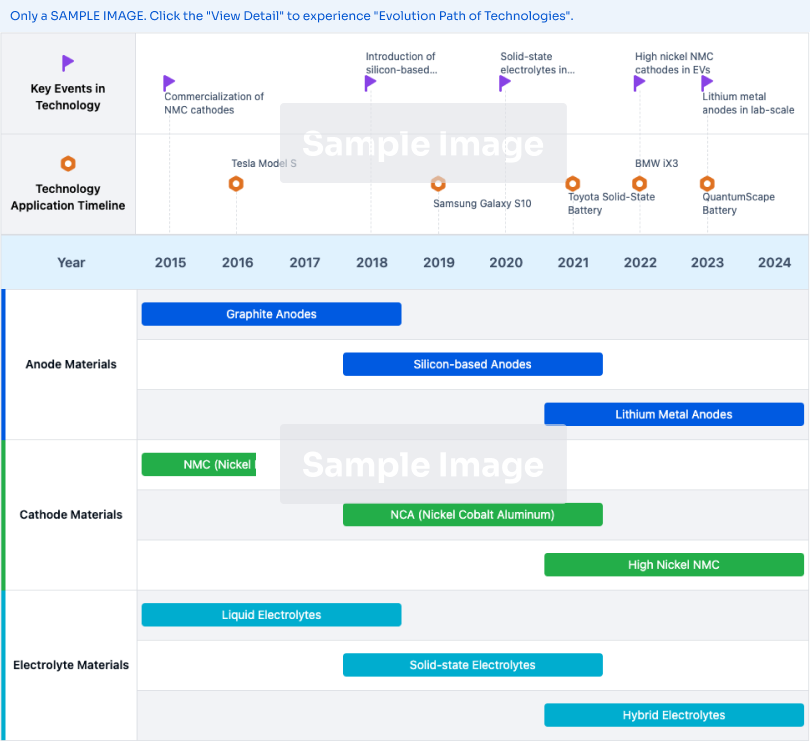
Current AI and ML Solutions
01 Healthcare AI and ML
Covers AI and ML techniques for medical diagnosis, disease prediction, treatment recommendation, and patient monitoring. Models analyze medical images, patient records, and sensor data to support clinical decision-making.- Healthcare AI and ML: Covers AI and ML techniques for medical diagnosis, disease prediction, treatment recommendation, and patient monitoring. Models analyze medical images, patient records, and sensor data to support clinical decision-making.
- Education AI and ML: Focuses on AI and ML for e-learning platforms, personalized learning experiences, student performance evaluation, and curriculum optimization. Models analyze student data, adapt learning materials, and provide intelligent tutoring systems.
- Business Intelligence and Marketing AI and ML: Covers AI and ML for customer preference prediction, marketing strategy optimization, stock market forecasting, and supply chain management. Models analyze large datasets to uncover insights and patterns for informed decision-making.
- AI and ML Infrastructure and Deployment: Focuses on infrastructure and deployment aspects of AI and ML systems, including hyperscale computing resources, continuous integration and delivery pipelines, performance evaluation, and model validation. Covers technical aspects of building and maintaining AI and ML systems at scale.
- Computer Vision and Image Analysis AI and ML: Covers AI and ML techniques for computer vision and image analysis tasks, such as object detection, image classification, and visual output generation. Models process and interpret visual data for applications like security, surveillance, and medical imaging.
02 Education AI and ML
Focuses on AI and ML for e-learning platforms, personalized learning, student performance evaluation, and curriculum optimization. Models analyze student data, adapt content delivery, and provide intelligent tutoring.03 Marketing and Customer Engagement AI and ML
Covers AI and ML for targeted advertising, customer segmentation, preference prediction, and personalized recommendations. Models analyze customer data, behavior patterns, and market trends to optimize marketing efforts.04 Business Intelligence and Decision Support AI and ML
Involves AI and ML for data analysis, predictive modeling, and optimization. Models help organizations gain insights from large datasets, identify patterns, and make informed decisions across various domains.05 AI and ML Infrastructure and System Development
Covers development of AI and ML systems, including hardware and software infrastructure, model training and deployment, performance evaluation, and continuous integration and delivery. Includes explainable AI, model validation, and ensemble policies.
Key Players in AI and ML Industry
Huawei Technologies Co., Ltd.
International Business Machines Corp.
Core Innovations in AI and ML
- The utilization of machine learning techniques to analyze and enhance the performance of faculties. this approach offers a novel and advanced method for evaluating faculty performance, which is a critical aspect in educational institutions. by leveraging artificial intelligence and machine learning algorithms, the system can provide more reliable and data-driven insights into faculty performance, leading to better decision-making processes and ultimately improving the overall quality of education.furthermore, the patent solution stands out for its comprehensive analysis of the ongoing industrial efforts in the machine learning landscape. by conducting a patent review and analysis, the system not only identifies key players and trends in the field but also delves into technological developments across various industries. this in-depth understanding of the industrial r&d efforts in machine learning technologies provides valuable insights for researchers and practitioners, enabling them to stay abreast of the latest advancements and innovations in the field.
Future Directions in AI and ML Research
- Multimodal AI Systems
- Trustworthy and Ethical AI
- Continual Learning and Open-Ended AI