Research on applying reinforcement learning for adaptive control in complex systems - Eureka
Reinforcement Learning in Adaptive Control: Background and Goals
The research aims to develop RL-based algorithms and frameworks that can continuously learn and adapt to changing system conditions, enabling real-time adjustments and optimizations. This could lead to improved efficiency, robustness, and resilience in various complex systems, such as manufacturing processes, energy grids, transportation networks, and cyber-physical systems.
Market Demand for Adaptive Control in Complex Systems
- Market Size and Growth
The global market for adaptive control systems in complex environments is rapidly expanding, driven by the increasing complexity of industrial processes, infrastructure systems, and emerging applications like autonomous vehicles and smart grids. - Key Application Areas
Major application domains include manufacturing, energy systems, transportation networks, robotics, and environmental monitoring, where adaptive control can optimize performance, improve efficiency, and enhance resilience. - Industry Trends
There is a growing demand for intelligent and self-optimizing control systems that can handle dynamic conditions, uncertainties, and disturbances. This trend is fueled by the adoption of Industry 4.0, IoT, and digital transformation initiatives across various sectors.
Current State and Challenges of Reinforcement Learning in Control Systems
- Reinforcement Learning Adoption
Reinforcement learning (RL) techniques are gaining traction in control systems, but adoption is still limited due to challenges in real-world deployment. - Complexity and Uncertainty
Complex systems with high dimensionality, nonlinearities, and uncertainties pose significant challenges for RL algorithms to learn optimal control policies. - Safety and Robustness Concerns
Ensuring safe exploration, stability, and robustness during the learning process is crucial, especially in safety-critical control applications. - Data Efficiency and Sample Complexity
RL algorithms often require large amounts of data and interactions with the environment, which can be impractical or expensive in many control systems. - Reward Shaping and Exploration
Designing appropriate reward functions and exploration strategies to guide the learning process effectively remains a significant challenge.
Evolution of Adaptive Control and Reinforcement Learning Technologies
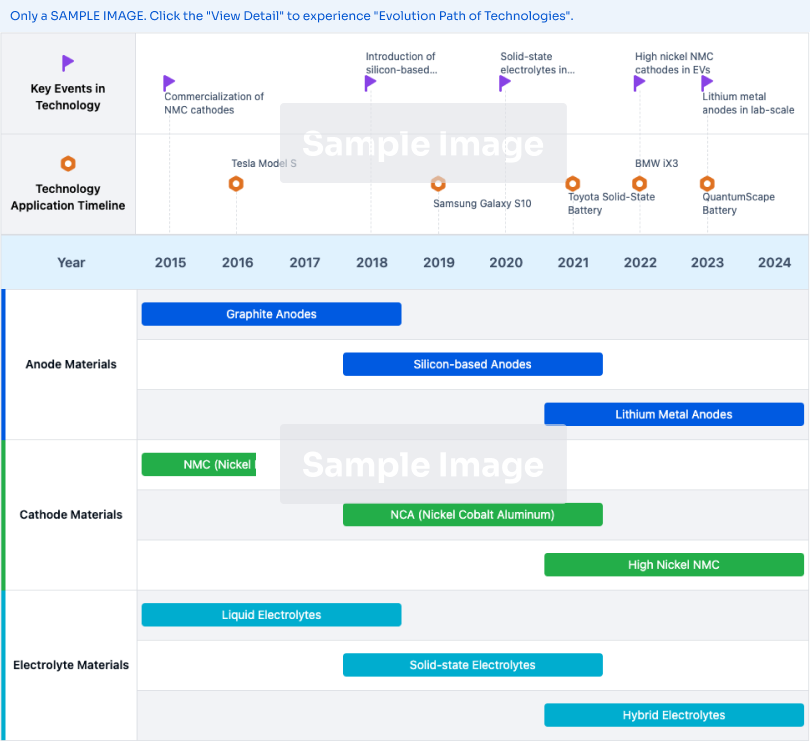
Existing Solutions for Adaptive Control Using Reinforcement Learning
01 Autonomous Systems Adaptive Control
These inventions utilize reinforcement learning techniques for adaptive control of autonomous systems like robots, vehicles, and UAVs. The algorithms learn optimal control policies for tasks like trajectory tracking, path planning, and stable flight control, adapting to changing environments or conditions.- Autonomous Systems Adaptive Control: These inventions utilize reinforcement learning techniques for adaptive control of autonomous systems like robots, vehicles, and UAVs. The algorithms learn optimal control policies for tasks like trajectory tracking, path planning, and stable flight control, adapting to changing environments or conditions.
- Industrial Processes Adaptive Control: These inventions apply reinforcement learning for adaptive control of industrial processes like motor control, signal control, and resource management. The algorithms optimize control parameters or policies for efficient and stable operation, adapting to changes in operating conditions or system dynamics.
- Energy Systems Adaptive Control: These inventions relate to using reinforcement learning for adaptive control of energy systems like hybrid electric vehicles and energy management systems. The algorithms optimize energy usage and distribution, adapting to varying operating conditions and energy demands.
- Meta-Reinforcement Learning for Adaptive Control: These inventions involve meta-reinforcement learning techniques for adaptive control. The algorithms learn a meta-policy or meta-controller that can quickly adapt to new tasks or environments, reducing the need for extensive retraining or fine-tuning for each new scenario.
- Adaptive Exploration for Reinforcement Learning: These inventions focus on adaptive exploration strategies for reinforcement learning algorithms. The methods adaptively adjust the exploration rate or strategy based on learning progress or task complexity, leading to more efficient and effective learning.
02 Traffic Signal Systems Adaptive Control
These inventions apply reinforcement learning for adaptive control of traffic signal systems. The algorithms optimize signal timing and coordination based on real-time traffic conditions and patterns, aiming to improve traffic flow and reduce congestion.03 Energy Management Systems Adaptive Control
These inventions use reinforcement learning for adaptive control of energy management systems, such as hybrid electric vehicles and power systems. The algorithms optimize energy usage and distribution, adapting to factors like driving conditions, battery state, and power demand.04 Robotic Systems Adaptive Control
These inventions apply reinforcement learning for adaptive control of robotic systems like manipulators, legged robots, and unmanned ships. The algorithms learn optimal control policies for tasks like grasping, walking, and trajectory tracking, adapting to environmental or task condition changes.05 Meta-Reinforcement Learning for Adaptive Control
These inventions relate to meta-reinforcement learning techniques for adaptive control. Meta-reinforcement learning involves learning a meta-policy or meta-strategy that can quickly adapt to new tasks or environments, reducing the need for extensive retraining.
Key Players in Reinforcement Learning and Control Systems
DeepMind Technologies Ltd.
International Business Machines Corp.
Core Innovations in Reinforcement Learning for Control Systems
- Integrating safety guarantees: by limiting the control policies to those that are predefined to be stable, the scheme ensures that the machine operates within a safe range, even when faced with uncertainties or disturbances.
- Global uncertainty characterizations: the scheme considers the entire controlled system, not just the dynamics or cost of the individual parts, when evaluating control policies. this allows for the consideration of a wider range of scenarios and provides a more comprehensive understanding of system performance.
- Continuous projection functions: the projection function used in this scheme is continuous, piecewise differentiable, and maps the raw control signal space to the stable control signal space. this allows for the use of a larger variety of sensor inputs and provides flexibility in control policy selection.
- Learning algorithms: by incorporating learning algorithms, such as back propagation, into the neural network training process, the scheme enables the neural network to adapt and improve its performance over time, even when faced with slightly different system dynamics or disturbances.
Future Directions in Adaptive Control with Reinforcement Learning
- Distributed Reinforcement Learning for Large-Scale Systems
- Safe Reinforcement Learning for Mission-Critical Systems
- Transfer and Meta-Learning for Rapid Adaptation