Hybrid Control Systems Integrating Model-Based and Data-Driven Approaches - Eureka
Hybrid Control Systems Background and Objectives
The key challenge lies in establishing a unified framework that can effectively combine the two approaches, addressing their respective limitations and exploiting their complementary advantages. This involves developing algorithms and architectures that can fuse the model-based control laws with data-driven learning and adaptation mechanisms, enabling robust and efficient control in dynamic, uncertain environments.
Market Demand for Hybrid Control Systems
- Growing Demand for Automation
Hybrid control systems are increasingly sought after to automate complex processes across various industries, reducing human intervention and improving efficiency. - Need for Adaptability
As manufacturing processes and operational environments evolve, there is a demand for control systems that can adapt to changing conditions through data-driven learning. - Improved Process Optimization
By combining model-based control with data-driven techniques, hybrid systems offer enhanced process optimization, leading to better resource utilization and cost savings. - Handling Uncertainties
Many industrial processes involve uncertainties that are difficult to model accurately. Hybrid systems can leverage data-driven approaches to handle these uncertainties more effectively. - Emerging Applications
Emerging applications like autonomous systems, smart manufacturing, and cyber-physical systems are driving the demand for hybrid control solutions that can integrate diverse data sources and models.
Current State and Challenges of Hybrid Control Systems
- Technology Maturity
Hybrid control systems are an emerging field, with ongoing research efforts to integrate model-based and data-driven approaches. While model-based control has been widely studied, the integration with data-driven techniques is still in its early stages. - Key Challenges
Challenges include handling model uncertainties, dealing with complex nonlinear dynamics, ensuring stability and robustness, and developing efficient algorithms for real-time implementation. Integrating heterogeneous data sources and addressing data quality issues are also critical. - Geographical Distribution
Research on hybrid control systems is being conducted globally, with major contributions from academic institutions and research labs in the United States, Europe, and Asia, particularly in countries with strong expertise in control theory and machine learning.
Evolution of Hybrid Control Technologies
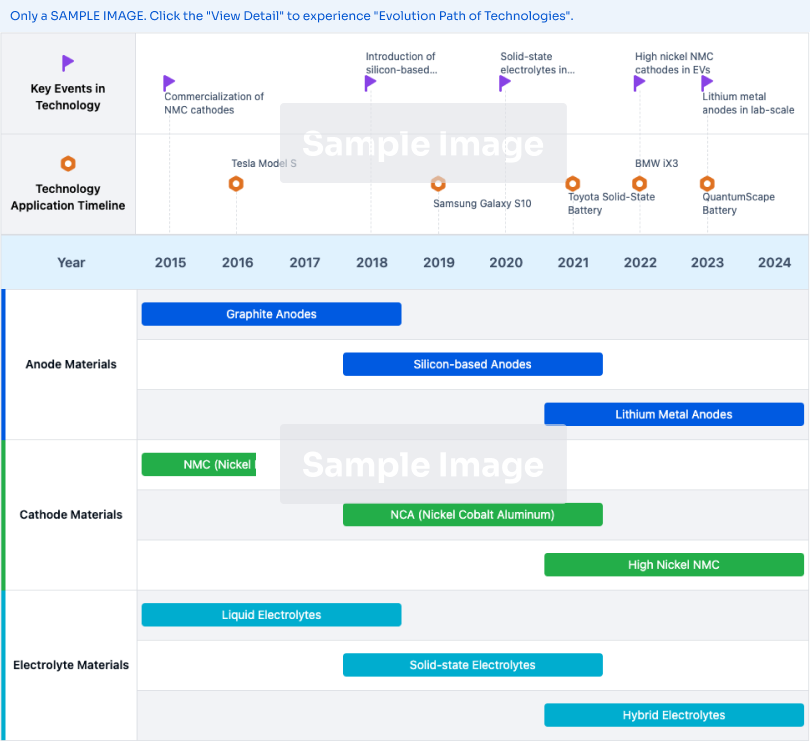
Current Hybrid Control Solutions
01 Hybrid Control Systems
These systems combine model-based and data-driven approaches to improve control performance and adaptability. The model-based approach provides a theoretical foundation, while the data-driven approach leverages real-world data to enhance capabilities.- Hybrid Control Systems: These systems combine model-based and data-driven approaches to improve system performance and adaptability, leveraging the strengths of both approaches.
- Hybrid Modeling Techniques: These techniques involve developing hybrid models that combine different modeling approaches, such as mechanistic, conceptual, and data-driven models, to capture the behavior of complex systems more accurately.
- Data and Model Integration Frameworks: These frameworks facilitate the integration of data from various sources and the integration of different models or modeling approaches, providing a unified platform for combining and managing heterogeneous data and models.
- Hybrid Vehicle and Robotics Control Systems: These systems integrate model-based and data-driven approaches for controlling vehicles, robots, or other mobile systems, improving navigation, decision-making, and overall system performance in dynamic environments.
- Hybrid Anomaly Detection and Fault Diagnosis: These systems combine model-based and data-driven techniques for detecting anomalies, faults, or abnormal behavior in complex systems, improving the accuracy and robustness of anomaly detection and fault diagnosis.
02 Hybrid Modeling and Simulation
These approaches integrate multiple modeling techniques, such as physical, conceptual, and data-driven models, to accurately represent complex systems for simulation, analysis, and control purposes.03 Hybrid Digital Twin Models
These models combine physical models, sensor data, and machine learning techniques to create virtual representations of real-world systems for predictive maintenance, optimization, and control.04 Hybrid Vehicle Control Systems
These systems integrate model-based and data-driven techniques to optimize performance and energy efficiency of electric and hybrid vehicles, adapting to varying driving conditions and user behavior.05 Data and Model Integration Frameworks
These frameworks provide methods and tools for integrating data from multiple sources and combining different types of models, enabling the creation of hybrid systems that leverage both model-based and data-driven approaches.
Key Players in Hybrid Control Systems
Hyundai Motor Co., Ltd.
Robert Bosch GmbH
Core Innovations in Hybrid Control Systems
- Integrating data-based and physics-based models to create a hybrid system for real-time controls and recommendations
- Leveraging data-driven models and physics-based models to optimize enterprise applications like HVAC, power generation, energy efficiency, etc.
- Developing a predictive model built on fundamentals to balance bias and variance, ensuring consistent and interpretable outcomes
Future Directions for Hybrid Control Systems
- Reinforcement Learning for Hybrid Control
- Hybrid Physics-Informed Neural Networks
- Hybrid Adaptive Control with Online Learning